Maximizing production potential using predictive maintenance
Today, many maintenance strategies of manufacturing companies rely on reactive approaches to machine failures or schedule-based maintenance performed by personnel. Sometimes, this imposes significant time and financial burdens for manufacturers. But what if machines never failed unexpectedly? And maintenance was more proactive rather than reactive? With the large volumes of data from production processes manufacturing companies acquire today, this could soon become a reality. Machine learning and predictive monitoring have the ability to transform the maintenance strategies of manufacturing companies - and we’ve done the research.
Motivations: The benefits of anomaly detection
Initiated and led by data management and analysis student Felix Boltshauser, we’ve explored the application of anomaly detection to establish a connection between analytical data and future failures, motivated by these benefits:
● Enhancing production efficiency
By embracing predictive
maintenance,
manufacturing companies can become more
data-driven
, reducing the need for reactive or schedule-based
maintenance. This will enhance overall production efficiency.
As a first step, the correlation between abnormal patterns in
data and tool failures must be assessed.
● Promoting sustainable manufacturing
Implementing predictive
maintenance aligns with UN Sustainable Development Goal 8
since it leads to reduced resource and material footprint,
economic gains through minimized machine downtime, and
improved operator safety.
In addition to industrial and societal gains, we addressed current scientific problems and delivered news value by focusing on:
● Handling imbalanced datasets by investigating how to effectively handle the imbalance between failure and normal working conditions in manufacturing machines.
● Exploring new machine learning models by investigating two models that have not been previously studied in the context of manufacturing data anomaly detection.
● Using a standardized data vocabulary to distinguish pre-failure machine states from normal working conditions, with potential application across multiple machines and production processes.
Findings: The potential of predictive maintenance
Through trials on multiple production processes using the standardized data collection methodology MTConnect, we looked into the distribution of anomalies prior to failure and during regular machine operation. Here’s what we found.
- There were more unusual events happening right before tools failed, which emphasizes the potential to use a predictive maintenance strategy.
- Some tool failures demonstrated abnormal behavior as early as seven production cycles prior to the failure, while others exhibited indications within the same production cycle.
- One of the newly proposed models that were used outperformed a current state-of-the-art methodology in effectively differentiating pre-failure and normal states based on anomalies.
- Both models applied exhibited excellent time and memory reliability, reducing the dependency on high computational power.
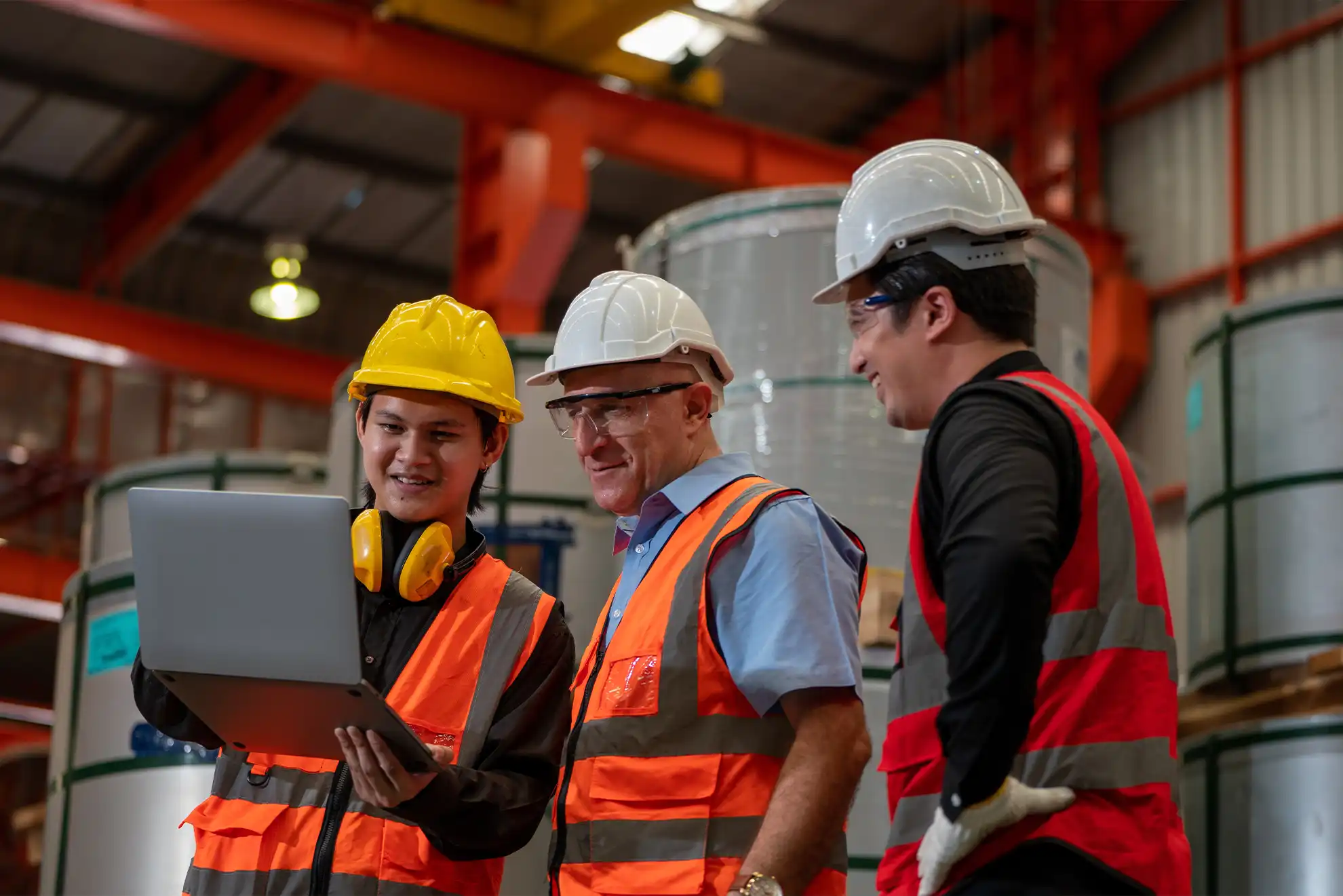
What does this mean for manufacturing companies?
Our research advances predictive maintenance methodologies and opens new avenues for improved productivity and sustainability in the manufacturing industry. What we’re trying to say is that maintenance strategies are evolving from reactive to proactive ones. A predictive maintenance strategy can benefit your manufacturing company by making production more sustainable and efficient. Using advanced technology, such as machine learning, your machines can effectively detect anomalies, prevent failures, and reduce machine downtime, which is essentially what will transform production processes.